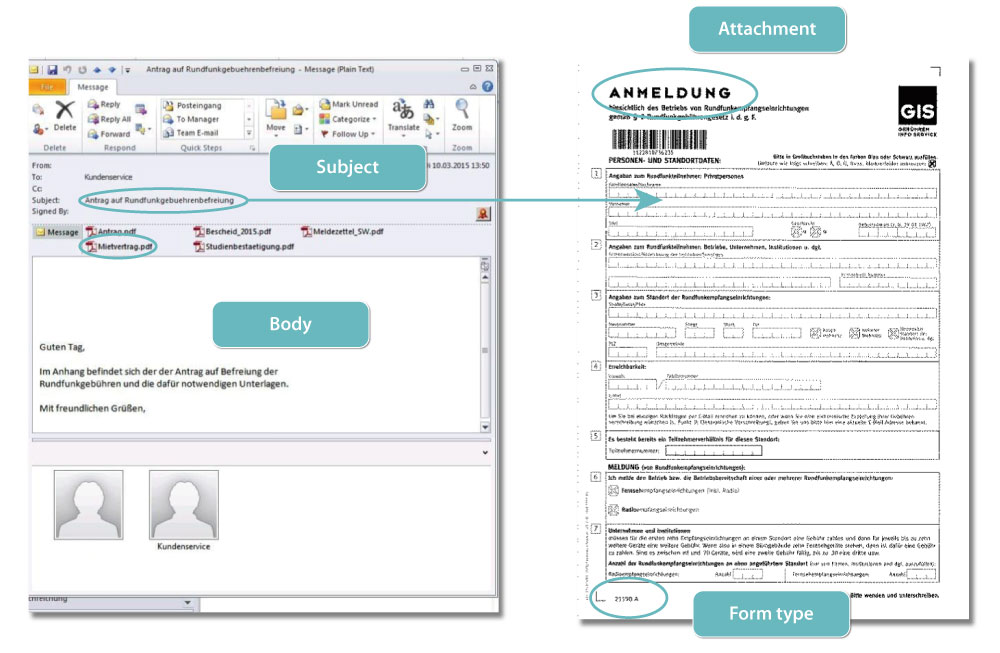
Product Description
Papyrus Classify is a self-learning module for classification of documents that offers a broad range of possibilities for application in the field of automated sorting and distribution of electronic documents, fax and paper mail. The purpose of Papyrus Classify is to enable an automated process of judging incoming data according to selected criteria, sorting them into freely definable categories - thereby making information actually accessible for the company - and forwarding them accurately to those who are in charge of the issue. A typical application would be automated pre-sorting of incoming electronic mail, e.g. matching e-mail topics with the assigned company departments (Accounting, Ordering, Support, etc.) or with specific persons (customer care, etc.). It is also perfectly suitable for automatic classification of text print in different languages according to language criteria.
Features
Operating mode
Papyrus Classify applies the knowledge of rules that it acquired through training to unknown documents during the classification process. For each document, the program calculates the probability of the print fitting into each category. Print gets allocated in the category which features the highest probability, as long as a pre-defined minimum probability is met in the first place.
Subsequently the documents are forwarded to their assigned destination (branch office, department, person, etc.).
Documents with an all-too-low probability for classification are placed in a so-called Reject Directory, from which the administrator can assign them to the right category via mouse click. If desired, these texts can be put to use for further training of the system, so Papyrus Classify can constantly learn and extend its knowledge base while fully delivering on its everyday chores. The user returns potentially misclassified texts to the administrator, who in turn proceeds with these just as with low-probability cases.
Papyrus Classify runs mainly in the background. For manual intervention by an administrator or user, a clear, simple and intuitive graphical interface is available.
Functions/Highlights
Self-learning system
Rules necessary for the automatic assignment of documents do not have to be specified, updated or adjusted to every change of the requirements by an administrator. Instead, the system is provided with a certain number of documents for each document class (typically two or three dozen) for training purposes. From this input Papyrus Classify learns the rules, according to which classification is effected, all by itself.
Easy re-training of fully functioning system
Papyrus Classify, while running in real-time mode, can constantly be fine tuned based on the documents that could not be assigned correctly. This ensures continuous, long-time optimizing of the system and enables flexible adjustment of the rules according to changes in document classes.
Exception handling
Documents which could not be assigned to a class unequivocally are separated and presented to the administrator for further assessment. These documents are then sorted in manually and, in the process, can be used for re-training the system.
Assigning a document to several classes
Multi-Stage Class Hierarchy
This enables a multi-level pre-sorting of documents; e.g. a document of the class “Invoice” is assigned to a sub-class “Supplier A”.
Prerequisites
Technical Prerequisites
Hardware
- Pentium IV or higher
- 512 MB RAM (1 GB recommended)
- 2GB HD
- per each Recognition-module there should be a Reco-server designated for better performance
Software
- Papyrus Capture base system
Dataformats
Supports a large number of file formats, for instance Text (DOS, ANSI, Unicode, UUEncode, MIME), Microsoft Word (Version 6.0 or higher), Microsoft Excel (Version 2.x or higher), HTML, RTF, WordPerfect (Version 6.0 or higher), Wordstar, Microsoft Works, Powerpoint, Lotus WordPro, Microsoft Outlook Mail Format, AmiPro.
Organizational premises
Before Papyrus Classify is implemented the required document categories have to be defined. With a number of sample documents (20-30) for each category the system is trained in order to learn the specific properties of each document class. New categories can also be added easily and conveniently while the system is in full operation.